By Trisalyn Nelson
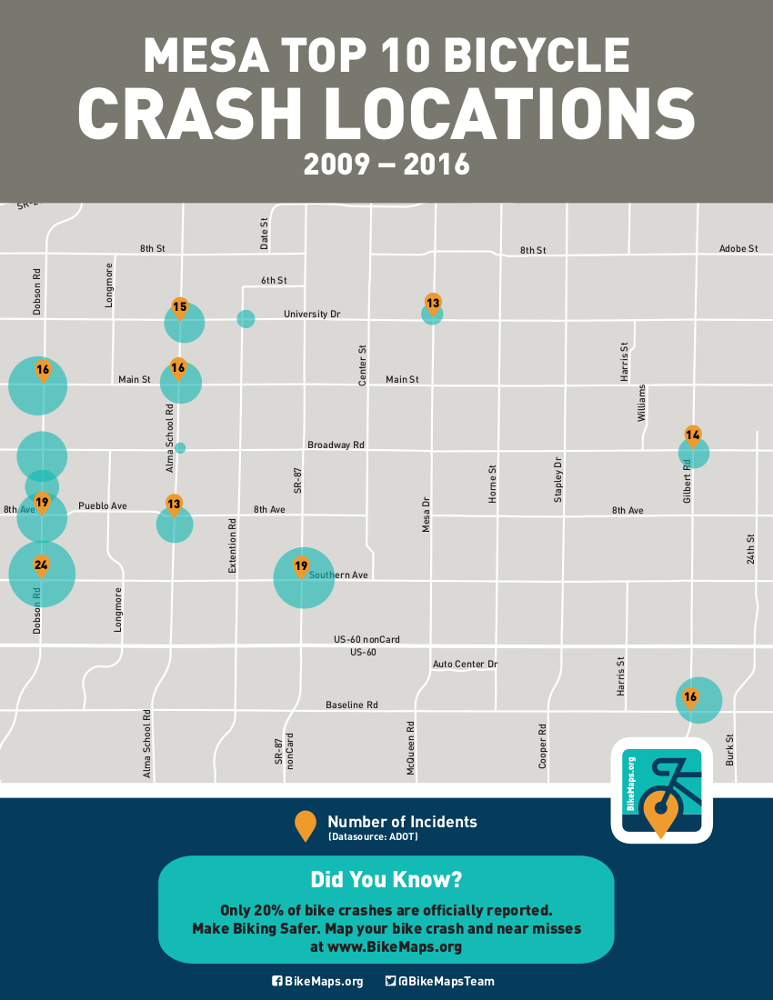
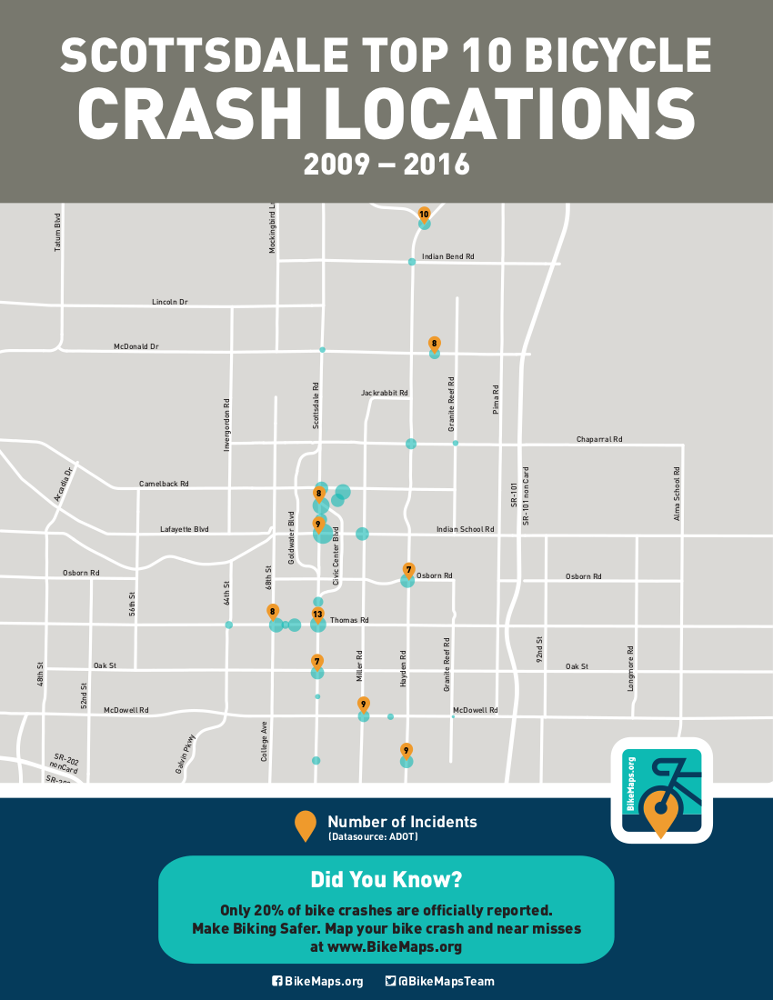
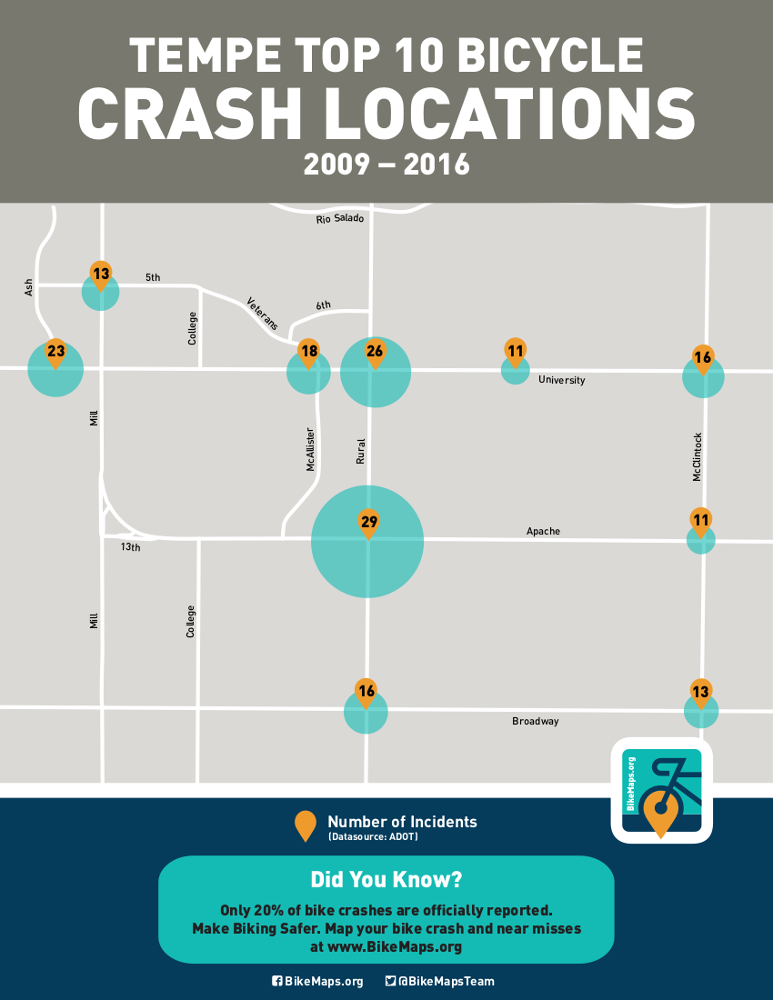
By Trisalyn Nelson
by Jaimy Fischer
Studies on bicycling safety have typically relied on data generated through traditional reporting systems such as police and insurance reports. These data are lacking important details that would allow for more robust studies on bicycling safety. Even more important, 70-80% of bicycling incidents are not recorded(1), either because police/insurance are not initiated, the incident was a near miss, or because the incident was bicyclist-only and did not involve a motor vehicle.
BikeMappers know that we collect quite a few variables on the incident details, some of them mandatory and others voluntary. But what do we do with all of them?
All the variables that we collect are designed to support better research on the circumstances that lead bicycling risk and injury.
Below is a sample of some of the variables that we collect.
Crash or near miss details | Conditions | Personal details |
---|---|---|
When was the incident? | What were the road conditions? | What is your year and month of birth? |
Select date and time | Dry | Select year and month |
What type of incident was it? | Wet | No response |
Collision with stationary object or vehicle | Loose sand, gravel, or dirt | Please select your sex |
Collision with moving object or vehicle | Icy | Male |
Near collision with stationary object or vehicle | Snowy | Female |
Near collision with moving object or vehicle | I don’t remember | No response |
Lost control and fell | No response | Other |
What sort of object did you collide with? | How were the sightlines? | Do you bike at least once a week? |
So how can we use BikeMaps.org data to evaluate injury and safety?
One thing we can do is take variables collected through BikeMaps.org reports and use them in predictive modelling. Recently we used a random forest classifier to model predictors of injury in Victoria, BC, Canada.
What is a random forest classifier?
A random forest classifier is an extension of decision tree learning. A decision tree looks like a flow chart, where each internal node is a test on a variable, aka predictor, and each branch represents the outcome of the respective test. Each leaf (terminal node) is a class label, or decision. Shown below, as a predictor drops down the tree it is grouped into smaller and smaller datasets based on its level (for example yes or no). The end result illustrates how predictors are related to an outcome, and the tree splits demonstrate the best partitions of the data.
In the simple example below that uses a dataset on the Titanic, passenger survival is the outcome that is modelled. The blue diamonds are internal nodes that represent a predictor test, and the branches are the data splits. The leaves (terminal nodes) are the decision, in this example, whether the person survived or died when Titanic sunk.
A random forest classifier uses an average of a collection of many decisions trees in its final decisions. Instead of relying on a single decision tree, in a random forest you can grow, say, 1000 trees (a forest!). In each tree of a random forest, only a random sample of predictors and their levels are used to model the outcome (i.e. 10%). In a forest of 1000 trees, the random sampling process is repeated 1000 times, creating 1000 individual decision trees, and in the end, all of the trees “vote” on the top predictors of an outcome. Once the forest is grown, you can then look at the relationship between the predictors and the outcome.
We used a dataset of 111 collisions and 234 near misses for Victoria. This dataset contained 23 predictors, and each predictor had multiple levels. We modeled for the outcome of injury based on the predictors below.
Note: Our dataset was not quite large enough to break down injury into specific categories as collected in BikeMaps.org reports, instead, we grouped data into a simple injury/no injury outcome. In the future, we might have enough data to model injury severity, that is, what are the predictors that would require a hospital emergency visit or stay vs a doctor vs no treatment.
Predictor | Mandatory (m) or voluntary (v) | Predictor levels |
---|---|---|
BikeMaps.org incident details | ||
Were you injured (outcome) | m | Yes | no |
What sort of object did you collide or nearly collide with? | m | Vehicle | person | infrastructure | other |
What was the purpose of your trip? | v | Commute | exercise or recreation | personal business | social reason |
BikeMaps.org incident conditions | ||
What were the road conditions? | v | Dry | wet | loose sand or gravel | icy | snowy | don’t remember |
How were the sightlines? | v | No obstructions | view obstructed | glare or reflection | obstruction on road | don’t remember |
*Were there cars parked on the roadside? | v | Yes | no |
*Where were you riding your bike? | v | Street with no bicycle facility | on a local street bikeway (bike route) | on a painted bicycle lane | on a cycle track | on a multi-use path |
*What was the terrain like? | v | Uphill | downhill | flat | don’t remember |
How were you moving? | v | Heading straight | turning left | turning right | don’t remember |
BikeMaps.org incident personal details | ||
Please select your sex | v | Male | female | other |
Do you bike at least once per week? | v | Yes | no | don’t know |
Were you wearing a helmet? | v | Yes | no | don’t know |
Additional predictors collected via BikeMaps.org, not submitted by citizen mappers | ||
**Precipitation | -- | Rain | none |
**Visibility | -- | Daytime | dawn/dusk | dark |
Time of the incident | -- | AM peak | PM peak | off peak |
Other predictors based on past research collected from additional sources | ||
Road classification | -- | Arterial | collector | local | off-street path |
Road width | -- | 4-28 meters |
Road speed | -- | 20 km/hr| 30 km/hr| 40km/hr| 50 km/hr |
Slope classification | -- | flat | > 1° (perceived slope) |
Is the roadway a truck route? | -- | Yes |No |
Did the incident occur at an intersection? | -- | Yes |No |
Bicycle ridership | -- | High |Medium | Low |
*to ensure modelling was as accurate as possible, these answers verified with external datasets
** Precipitation is collected and applied to BikeMaps.org reports via a web scraping application. Snow, sleet etc. would be included had those conditions occurred in Victoria at the time of a given report.
Results
In order, the top 5 predictors of injury were:
Below are partial dependence plots, which are an output from the random forest model. These plots are good for visualizing how each predictor and its different levels influence the outcome we are predicting with a random forest classifier. The plots are read as the increasing or decreasing probability of a classification with respect to the different values of a given predictor variable while holding all other predictors constant. For example, below depicts the predictor “What sort of object did you collide with?”, and the classification we are interested in is injury. You can see that, according to our model, collisions with the roadway, train tracks, and a vehicle open door have a higher likelihood of an injury classification.
1) Overall, the strongest predictors of injury were bicyclist-only crashes (including falls), and doorings. Collisions with infrastructure such as the roadway (i.e. as a result of avoidance or slipping on the road surface), a pothole or train tracks are examples that were reported by citizen mappers. The “other” category refers to reports that involved an object not listed in the incident pull-down menu. For example, one report indicated that the bicyclist collided with an unusual hazard on the road.
2) Next in predicting injury was the type of bicycle facility that the person was riding on, where painted on-street bicycle lanes and multi-use paths had a higher influence on an injury classification. Research has shown that these types of infrastructure have higher risk than other types.(2)
3) Estimated ridership at crash site.
4) Road conditions.
5) Trip purpose.
Reflections
Bicyclist-only crashes often result in injury, but are rarely captured in traditional reports(1,5). Our analysis results echo past research using surveys, interviews, or hospital administrative data (all time-consuming methods), which identify road surface features and conditions along the route as risk factors in bicyclist-only crashes(2,5). Similarly, in crashes involving other road users, our results mirror research that demonstrates higher risk on infrastructure such as multi-use paths, painted bike lanes and major roads with parked cars compared to bicycle-specific infrastructure(2). Our results also highlight novel findings on the importance of specific trip characteristics such as trip purpose and sightlines.
The findings in this analysis ultimately show that citizen science data can be complementary to bicycling safety research: specifically, that BikeMaps.org reports have the potential to address gaps in safety data and provide more detailed information on the circumstances of bicyclist crashes.
We have provided another method for analyzing BikeMaps.org reports. We used a random forest classifier as a novel approach to predictive modelling on the growing BikeMaps.org dataset, and demonstrate how BikeMaps.org data can help to address gaps in traditional reporting. We can take a closer look at top predictors by applying spatial analysis techniques to identify where top predictors are clustered. For example, is a there a specific area where bicyclist-only crashes are occurring more frequently? Thus, we can investigate unique results for different study areas. Results can be used by advocates, researchers, planners and other local stakeholders who are working to improve bicycling safety and increase ridership.
We look forward to improved modelling opportunities as more incidents are mapped!
References
by Tyler Stewart (BikeBridge Cycling Association)
Just like a tough uphill climb, sometimes you have to focus on the struggle one pedal crank at a time. Here in Lethbridge, it’s been a slow and steady grind but it seems like we’re finally starting to gain traction.
Like most urban centres, the windy city of Lethbridge (population nearing 100,000) was designed around the motor vehicle, not the bicycle. But a small group of dedicated folks have pushed forward to ensure the velocipede isn’t completely forgotten in its role to build a healthy, happy community.
Jane's Ride 2016
BikeBridge Cycling Association came together in 2010, when the City of Lethbridge had proposed a new cycling bylaw that included mandatory helmet regulations, and lumped in cyclists with all other “personal conveyance devices” such as rollerblades, skateboards and wheeled skis (does anyone still use those things?!). The proposed bylaw was dumped, but it’s taken many years to make progress on the bigger issues finally addressed in the recent Cycling Master Plan. Though far from perfect, it outlines an infrastructure network that will encourage safer cycling throughout the city to be developed over the next 20 years.
Bike in Film Night
While the lengthy timeline is frustrating (as is the lack of focus on education and awareness), BikeBridge has remained focused on developing the cycling community through a variety of events and initiatives to bring cyclists together. During the 2016 Tour of Alberta stage hosted in Lethbridge, a number of events like bike rodeos, cycle concerts and a bike-in film night helped promote the social aspect of cycling within the community. In 2017 we hosted a winter cycling film screening and held our second annual Jane’s Ride, a fun and informative historical cycling tour around town. We were also thrilled to participate in the BikeMaps.org initiative to gather more data around cycling hazards and incidents, which will hopefully lead to better designs around the upcoming infrastructure developments.
Bike Concert
This year, we’re looking to step it up a notch after receiving funding to host Lethbridge’s first-ever Bike Week program of events and activities. With group rides, workshops, another bike-in film festival and more fun ways to get out and ride, we’re hoping to foster more riders into getting out to “put some fun between their legs” this year in Lethbridge. In addition to reducing pollution and congestion on the roads, and being a great way to keep fit and have fun, BikeBridge is focused on the positive social role of cycling in our community.
Getting neighbours out beyond their front door to cycle together and learn from each other’s differences will hopefully create a more inclusive and welcoming city – one revolution at a time.
Second annual Jane's Ride (2017)
Keep in touch with us through our website at bikebridge.ca, on Twitter @BikeBridge or at Facebook.com/groups/bikebridge
By Trisalyn Nelson
Official bike crash reports account for 30-40% of all incidents. We used Arizona Department of Transportation (ADOT) bike crash data from 2009 to 2015 to map the 12 worst spots for bicycling in Tempe. But, there is a lot we still don’t know. Inform policy makers, advocates, and researchers about bicycling safety in your city! Map bicycling crashes, near misses, and hazards at BikeMaps.org.
By Moreno Zanotto
In the fall of 2016, Transport Canada assembled a working group to identify options for mitigating the safety issues surrounding cyclists and pedestrians (known as vulnerable road users, or VRUs), and heavy goods vehicles (HGVs). As a stakeholder in cycling safety and a Public Health Agency of Canada funded project, BikeMaps.org has provided input and feedback on the countermeasures being assembled.
Collisions with motor vehicles present the greatest safety threat to people cycling[1], and heavy vehicles (including trucks and buses) are overrepresented in fatal collisions with cyclists[2]. There are numerous interventions that can help improve road safety for VRUs (we’ll focus on cyclists in this post), although not all will be equally effective. For guidance on which interventions are most effective at protecting cyclists, we can look to the Netherlands, which has a large number of cyclists, yet has achieved some of the safest streets in the world. The Dutch strategy to improve road safety is known as Sustainable Safety. This philosophy treats the road system as inherently unsafe and regards humans as imperfect users of the system (we make mistakes and disregard rules)[3]. With that as the starting point, Sustainable Safety works to reduce the frequency and severity of collisions so that no one should die or be seriously injured on the road. Improving the subjective safety of vulnerable road users is also a key objective as feeling safe is very important to get more people to cycle for more of their trips. The results for the Netherlands has been impressive. Since the adoption of Sustainable Safety in the early 1990s, the number of traffic fatalities has decreased 45%[4].
Under Sustainable Safety, the road system (itself comprised of people, vehicles and the roadway) is organized around five principles[3]. Under these principles, we’ve listed five interventions that can systematically improve road safety for cyclists:
1) Homogeneity (of mass, speed, and direction)
Road users of greatly different mass and speed (e.g. trucks and cyclists) are segregated unless differences in speed can be reduced. A sustainably safe road system separates cyclists from car and truck traffic along major roads while mixing users under low-speed conditions on residential streets.
Segregate Modes on Major Streets (Build Protected Cycle Tracks)
Cyclists in a protected cycle track. People driving, cycling, and walking are segregated on this busier distributor road because car volumes and speeds do not allow for the safe mixing of different road user.
Continue Separation of Modes into the Intersection (Build Protected Intersections)
Protected intersections keep cyclists out of the truck’s blind spot and away from danger. Half of the fatal bicycle collisions with HGVs involve a side impact[1]—a problem easily avoided with safe junction design and signal timing. (Image credit: MassDOT)
2) (Mono)functionality
Roads are classified based on their function as either through roads for high volumes of fast-moving traffic or for local access to destinations and residences (plus a third type to connect through roads with access roads).
Separate Bicycle and Truck Routes
All residential streets are 30 km/h and employ some form of motor traffic calming, such as the ‘No Entry’ (except bicycles) sign on this local street in Utrecht. No long-distance car trips can be made on this street; it’s just for accessing the residences on this block. Mixed traffic conditions are possible because of low car speeds and volumes, otherwise, segregation of modes is necessary.
3) Predictability
Recognizable and consistent road designs make anticipating other road users’ behaviour (and where road users can be found) easier. The design should also encourage safer travel speeds.
Develop Recognizable Road Surfaces and Consistent Priority Procedures
Features such as elephants’ feet (indicating a cycle crossing), sharks’ teeth (give-way markings), and red asphalt (only for cycle paths) create a consistent look and feel for crossings where cyclists have priority. There is no ambiguity here—cyclists will be crossing and they have the right-of-way. Clearly recognizable and consistent design leads to predictable behaviour and safer streets.
4) Forgiveness
When road users make mistakes (and they will), the design softens the consequences.
Side-underrun Protection
In the event of a side collision between a cyclist and HGV, side-underrun protection prevents cyclists from falling under the truck and being run over by the rear wheels[5]. Side-underrun protection should be mandatory on all new heavy goods vehicles owned or operated in Canada. Lafarge Canada is voluntarily installing side guards and rails on its fleet.
5) State Awareness
Is the ability to assess one’s capacity, and that of others, to safely operate a vehicle or bicycle. This includes an altered state of mind from the use of alcohol and drugs, level of education and training to perform the driving or cycling task, and user competency (which varies based on experience and age). Road safety measures centred on regulations, education, and enforcement are organized under this principle.
People are vulnerable, they are fallible, and they don’t always follow the rules. Our road system must be designed to reflect this reality so that no one should die on the road. Separating incompatible road users in space or time, improving the predictability of road user behaviour, and making the road system more forgiving can help prevent collisions and reduce the severity of injuries should a collision occur. Except for side-underrun protection, the road safety improvements mentioned above are not specific to heavy vehicles and can yield safety benefits for all road users.
BikeMaps.org: Making Cycling Safer!
Incidents with HGVs are rare, but when they do occur, injuries are usually severe. As the only tool to systematically collect near-miss incident reports, BikeMaps.org can be used to identify danger spots before serious issues occur.
References